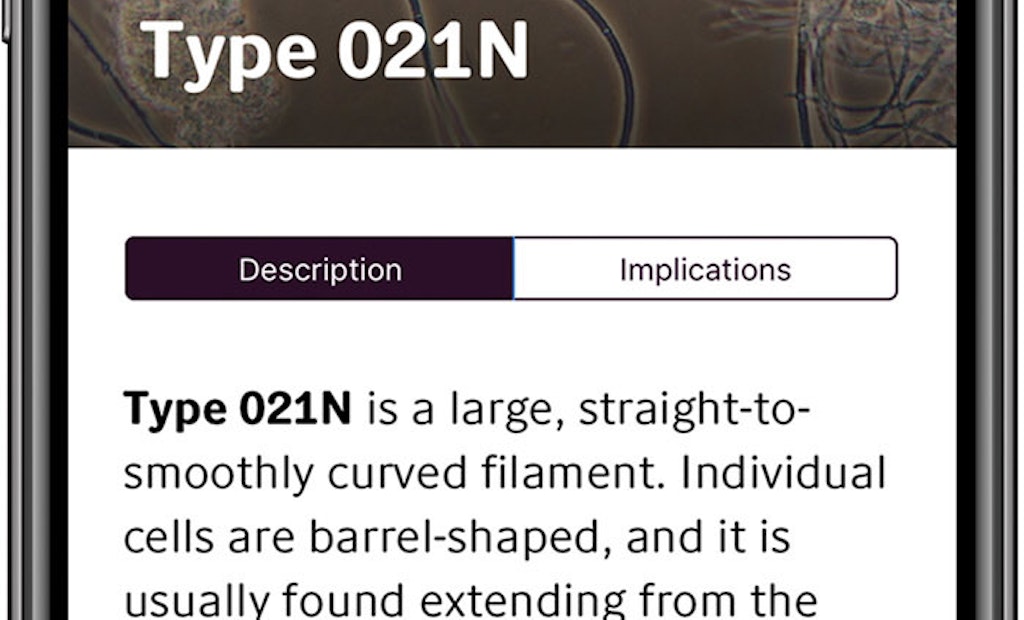
Plant Assistant reports on microscopic evaluation of filaments can be displayed on desktop computers or mobile devices.
Excessive filamentous bacteria can cause severe disruption in clean-water plants, most notably poor settling in final clarifiers.
When a filament issue arises, it’s essential to find the cause, and that starts with a microscopic study to determine the abundance and species of...